Research Data
The German Research Foundation considers research data to include:
measurement data, laboratory values, audiovisual information, texts, survey or observation data, methodological test procedures and questionnaires. Compilations and simulations can likewise constitute a key outcome of academic research and are therefore also included under the term research data. Research data in some subject areas is based on the analysis of objects (such as tissue, material, rock, water and soil samples, test specimens, installations, artifacts and art objects), so it must be handled just as carefully and consideration must be given to a technically adequate option for subsequent reuse whenever appropriate and possible. The same applies if software is required for the creation or processing of research data.
Source: Deutsche Forschungsdgemeinschaft (2023) [last access: 2023-05-23]
Examples of research data in Chemistry:
- simulations
- spectroscopy data
- chromatographic data
- experimental data
- electrochemical data
- database content
- models
- algorithms
- topographical data
- ellipsometric data
- interferometric data
- crystallographic data
- microscopy data
- software
Research Data Lifecycle
The research data lifecycle presents the steps necessary to map the process of a research project with regard to the research data. In particular, according to the UK Data Archive, the lifecycle includes the collection, processing, analysis, archiving, sharing, publishing, and re-use of research data.
Research Data Management
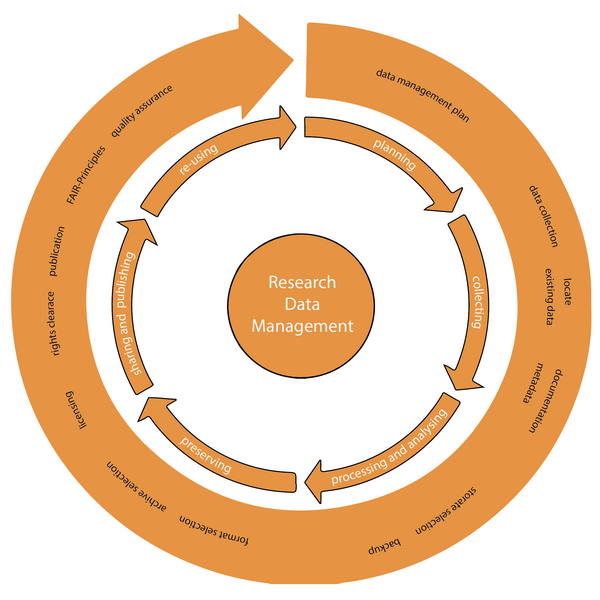
Following the research data lifecycle it's easy to define what research data management is: it is included in all steps of the research process. The central tasks of research data management are:
- Planning the handling of research data at the beginning of a research project and, if necessary, presenting the planned measures in funding proposals.
- Defining a folder structure and file naming conventions
- Documentation of research data and tagging with metadata
- Backup and long-term archiving of research data
- IT security and access control for research data
- Long-term archiving of research data
- Publication of research data
- Discovery and reuse of existing research data
- Consideration of data protection and copyright law when handling research data
FAIR Principles
The so-called FAIR principles comprise four goals: the findability, accessibility, interoperability and reusability of data. With the achievement of these goals, the sustainable reusability of research data is meant to be guaranteed.
The data and its metadata should be findable by humans as well as by machines, with basic machine-readable descriptive metadata enabling the retrieval of relevant data sets. In addition, the data and its metadata should be archived in such a way that they can easily be accessed, downloaded or used locally by humans and machines using standard communication protocols. The data should be available in a form in which it can be exchanged, interpreted and combined with other data sets by humans and machines in a (partially) automated manner. A good description of the data and its metadata is to ensure the reuse of the data for future research and the comparison with other compatible data sources. Proper citation of the data must be facilitated and the conditions for reuse need to be presented in a way that is comprehensible to humans and machines.
The main objective of the FAIR principles is to prepare the research data for humans and machines in the best possible way. This does not mean the data must be accessible without restrictions. Limited accessibility, for example due to the protection of data privacy, does not contradict the FAIR principles.